Alternative splicing acting as a bridge in evolution
Introduction
Introns play pivotal roles in eukaryotic evolution. The multitude functions of introns are still not fully understood. One important aspect of intron function is alternative splicing (AS). Fraction of alternatively spliced genes has been estimated to be 10% in C. elegans (1), 68% in human (2,3), 46% in Drosophila (4), and 24% for Arabidopsis thaliana and 33% for rice (5). The Basidiomycetous yeast Cryptococcus neoformans has 4.2% of AS (6). The dimorphic Basidiomycota yeast Ustilago maydis has 3.6% AS, corresponds to 26% of genes with introns (7). As much as 76% of multiexon genes from Trichoderma longibrachiatum have AS when probed with deep RNA sequencing technology (8). It is still a question whether abundant AS is a common phenomenon in primitive eukaryotes such as fungi.
In a survey of KOG clusters from 12 eukaryotic genomes, AS is more abundant in ancient genes and correlates with number of exons per gene (NEG) (9). Mechanistic studies have shown that introns can boost gene expression through every aspect of mRNA production (10-16). AS of eukaryotic genes is essential for generating diversity at both transcript and protein levels and for post-transcriptional regulations through nonsense-mediated mRNA decay (NMD) (17,18) that eliminates aberrant mRNA containing premature termination codons (PTC). NMD and AS have been thought to co-evolve (19). Short introns without in-frame stop codons from diverse eukaryotic lineages have evolved to avoid length of multiples of 3 ( 3n) presumably owing to their inability to be recognized by NMD when not spliced, or to be efficiently spliced (20). AS is used as one of the regulatory mechanisms in maintaining a delicate balance between pluripotency and differentiation in stem cells (21).
It is still not well understood how AS facilitates evolution. Here we used a novel EST assembly algorithm COMBEST to analyze AS and found that as much as 73% of the multiexon genes from fungi could have AS. Furthermore, AS is both ancient and conserved among fungal genomes. Finally we demonstrated that intron retention (RI) is an important mechanism for creating protein diversity.
Material and methods
Genome sequences
Genome sequences were all from the Joint Genome Institute (JGI). For clarity we used the JGI genome database name as abbreviations. Each database name is made of the first three letters of the genus name and the first two letters of the species name, and ends with a version number.
Source of EST sequences
For genome A. bisporus, there were 1,140,141 EST with average 221.6 nt generated by the JGI 454 pipeline. The EST came from several sources: vegetative casing soil, vegetative mycelium on compost-agar, and fruiting bodies.
Total 2,466,463 ESTs for A. carbonarius came from two pooled libraries CFSW and CFSO; both were generated with 454 sequencing machines. CFSW had 967,199 ESTs and was pooled from 25 samples by combination of 5 different time points with 5 different media that permit ochratoxin A production. CFSO had 1,499,264 ESTs and was pooled from 5 samples each from a different time points in YES medium that does not permit ochratoxin A production. In addition, there were 119 Sanger ESTs downloaded from NCBI (08-28-2009). The input ESTs had an average length of 401.8 nt.
For genome S. thermophile, we used 297,831,520 EST sequences with average length of 36.9 nt mainly produced by the Solexa sequencing platform with the shortest batch of 26 nt long. A subset of cDNA sequences were taken from four growth conditions: 13,262,122 EST from Glucose at 34 °C, 7,286,066 EST from Glucose at 45 °C, 7,793,637 EST from Alfalfa, and 13,116,200 EST from Wheat. There were 56,520 Sanger EST sequences.
The 355,237,724 EST sequences for L. bicolor were generated with three technologies. The average length for the EST was 57.4 nt. Of the EST sequences, there were 32,483 Sanger ESTs, and 181,289 generated from the 454 technology.
EST sequences, total 338,255,050, for A. aculeatus were generated from the 454 and Solexa platforms. The average sequence length was 78.2; this was longer than the average for S. thermophile and L. bicolor and was due to newer generations of Solexa machines generating longer sequences.
EST Assembly COMBEST Algorithm
The COMBEST algorithm was implemented in C++ with performance in mind, and the details will be published in a separate paper. Briefly, we first aligned EST sequences to genomic sequences with Gmap (22) or TopHat (http://tophat.cbcb.umd.edu/index.html) that produces alignments in SAM format. In the second stage we sorted the alignments on the genomic sequence and grouped overlapping alignments into congregations. Then, we computed the relationship matrix between alignments within the same congregation. There were four types of relationships between two alignments: compatible, contain, contained, and incompatible. The relationship between alignments was represented by a matrix of integers: 0 for incompatible, 1 for compatible, 2 for contain, and -2 for contained. This matrix represented a special graph between the alignments. Each node of the graph had the following properties: valance, color (white, gray, and black), parent node, in assembly, distance to root, and segment of model (the alignment). The valance was defined as 2*C+O, where C was containment relationship of this node against all other nodes, and O was compatible relationship of this node against all other nodes. It was used for picking the head node for starting each round of the assembly. To qualify as head node, the node must not be included in any EST assembly and has the highest valance of all non-assembled nodes. Each iteration of the algorithm was essentially the breadth first traversal of graphs from the head node. At each step of the traversal, the algorithm kept track of the base coverage profile (BCP) and the identifiers of ESTs. At the termination of each round of traversal one assembly (corresponding to one alternative isoform) was produced. At the end of the assembly process, from each congregation, one or more assemblies will be produced. One of the major challenges for constructing EST gene models was that there was significant transcription overlap (including antisense) of neighboring genes. This seemed to occur at low frequencies and could be a problem at high EST coverage. As part of the assembly process we also performed chimera resolution based on the presence of Dips and Drop-offs in the BCP, and this extra step proved to be very effective in breaking chimera of multiple gene models in the same assembly.
If an algorithm joins gene model fragments in all combinations, then there is a combinatorial effect. This could happen to some EST-based gene modeling algorithms. Some of the combined models may not be biologically relevant. One nice feature of this algorithm is that it suppresses combinatorial effect by picking the head-node for starting the assembly and prohibiting the head-node from being used more than once; although this will not complete eliminate the combinatorial effects.
Computation of intragenic expressed fraction (IEF)
The expressed fraction of each gene model is the sum of exon length divided by the genomic length of the same gene model. We used the average of this fraction from all gene models from each genome as IEF. It was an intragenic measure in that intergenic space was not accounted for because it was not easy to get an accurate estimate. This value was mainly used to normalize the EST coverage. Only full length COMBEST models were used for the computation of this value.
Computation of EST coverage
EST coverage was defined as the total mapped EST sequence divided by the non-gapped genomic length, then normalized (divided) by the IEF. The number of mapped EST was more meaningful because it excluded the influence of EST quality and completeness of genome assembly. This value was roughly the number of times each mRNA base is covered by EST on average (this is an under estimated since intergenic regions were not included in the coding fraction). However, IEF is comparable between genomes.
Measurement of overlapping transcription
A congregation is an intermediate entity of the COMBEST algorithm that includes all alignments overlapping each other in genomic space regardless of direction (Please see method section for more details). From each congregation COMBEST produces one or more assemblies. In sufficiently EST-covered loci, all the assemblies are full-length gene models. Before the chimera resolution step of the COMBEST algorithm, the various gene models from the same congregation are usually connected by Dips or Drop-offs in the BCP (data not shown) which indicated the low probability of transcription overlap. Gene in this study was defined as the models that were in the same direction and share significant coding regions. We used the fraction of congregations with more than one gene as the measure for transcription overlap. Similarly the fraction of genes from congregation of multiple genes was another measure of the extent of transcription overlap. The two values are closely related, but the later reflects the extent of congregation. Please note that we only used the set of full-length models for this computation, thus eliminated the influence of partial models.
Detection of antisense transcription
The focus of this study was not on antisense, but we needed to assess the extent of antisense. We define antisense models as a pair of models that overlaps each other on opposite directions with over 50% of either one without regarding the biological relevance. Furthermore, we did not distinguish between mRNA or regulatory RNA such as small interfering RNA (siRNA) or micro RNA (miRNA). Antisense is an explosive area of research and we are not going to elaborate on this.
Classification of major and minor alternative isoforms
The major isoform of the AS is defined as the gene models with the most number of EST in the corresponding gene models, all other isoforms are classified as minor isoforms. In very rare cases where two isoforms having the same number of EST sequences, we picked the one with the highest expression level. The relative expression level (abundance) of the minor isoform with respect to the major isoforms was computed as the minimum of the ratio between the BCP of the minor isoform compared to that of the major isoform.
Classification of AS
We distinguished eleven types of AS: alternative donor (AD), alternative acceptor (AA), cassette exons (CE), cassette exons with alternative donor (CE_AD), cassette exons with alternative acceptor (CE_AA), cassette exons with both alternative donor and acceptor (CE_ADAA), IR, alternative donor and alternative acceptor (ADAA), cassette exon or alternative acceptor at 3’-end (CEorAAend), mutually exclusive exons (ME), and other types. The type of AS refers to the minor isoform relative to the major isoform. RI was further divided into major, minor, and none. If the intron was retained in the major (or minor) isoform, then this was classified as major (minor) RI. If an intron never had an RI identified, then this intron was classified as No-RI and abbreviated as NRI in this paper.
Measure of ancientness
We used all proteins from three fungal genomes for blast search against non-fungal proteins (nr minus fungal proteins). Then we picked the best hits from each division: Viridiplantae, Metazoa, other Metazoa group, Archaea, Bacteria, and other unclassified taxa, followed by false positive hits elimination by comparing relative blast scores from different divisions. For example, if the score to Metazoa was 200 and to other Metazoa group was 900, we would drop the hits to Metazoa. After filtering, we consolidated the hits into three domains: Archaea, Bacteria and Eukaryota. A query can have eight possible hit patterns (Archaea+/− × Bacteria+/− × Eukaryota+/−), and the pattern with hits to all three domains (A+B+E+) is considered the more ancient. We divided the query proteins into AS and non-AS categories and compared the frequencies of the ancient hit pattern.
Exon alignment
We used a simple algorithm (unpublished algorithm from orpara.com) that aligns the exon structures of pairs of gene models by matching exons by their length while tolerating small differences of multiples of 3. Indel happens when exon from one gene is the sum of two or three exons from the other gene. The alignment qualities were classified into five categories: perfect, near perfect, having intron indels, partial alignment, and not aligned. In perfect alignment, all exons are aligned with minor length differences at the first or last exon. For near-perfect alignment, one or more internal exons are allowed to have small differences. If the alignment contains one or more insertion/deletion of introns, then we classify it as indel. Even though without exon alignment, some intron positions may be conserved in the context of protein sequence alignment.
Calculation of stopless intron bounds
We take 12 nucleotides from either ends of the intron bounds, with 3 nucleotides in the exon and 9 nucleotides in the intron from the non-redundant introns for each genome taken from the GeneCatalog track for each fungal genome. Then we tabulated the base frequency for each base and the total number of stop codons in all three phases. For phase 0, we picked the first and last two codons respectively in the intron. For phase 1 at 5’ end, we picked the last base from the preceding 5’ exon and first two bases from the intron as the first codon, followed by two codons in the intron; at the 3’ end, we counted three codons with the last codon composed of one base from the last base of the intron and two bases from the 3’ following exon. For phase 2 at 5’ end, we picked the last two bases from the preceding exon and one base from the intron as the first codon followed by two codons. The 3’ end of phase 2 had two codons with the last one comprises two bases from the intron and one base from the exon. The frequency of stop codon in each intron bound codon was computed by the product of the frequency of the three bases that make up the stop codon. This frequency is the expected stop codon. We also calculated the actual frequency of the occurrence of the stop codon. In most cases, the actual stop codon occurred with significantly lower frequency than the expected with very low binomial test P values. The overall stopless frequency was computed by multiplying the stopless frequencies of the two intron bounds for each phase. This was done for both the actual stop codon occurrence and expected. The difference was minimal for phase 0 and 2, but actual stop codon frequency is slightly higher than the expected for phase 1 (average 3.2% for five fungal genomes).
Results
The characteristics of genomic and EST sequences as well as abbreviations for six genomes used in figures and tables were detailed in the Methods section and Table 1. The EST coverage of the six genomes ranged from 3 to 834 respectively. We found significant transcription overlap between neighboring genes (Supplemental I; Figure S1), which explained why existing gene modelers produced abundant chimera models. Our COMBEST algorithm for assembling ESTs into gene models (Methods section for details) was designed to overcome these hurdles, and its quality performance had been validated at JGI.
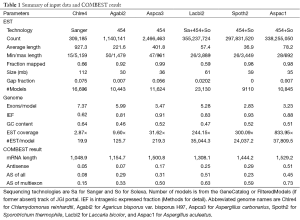
Full table
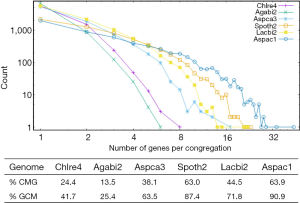
Majority of multi-exon genes have AS
The observed AS for a particular gene was proportional to the input EST coverage before reaching saturation after which the effectiveness of additional EST decreased exponentially (Supplemental II, Figure S2). Previously reported AS for fungal genomes ranged from 5% to 76%. Here, we observed 29%, 31%, 23%, 51%, and 45% for A. bisporus, A. carbonarius, S. thermophile, L. bicolor, and A. aculeatus; when normalized to multi-exon genes, the percentages became 33%, 50%, 59%, 63%, and 73%, respectively (Table 1). The C. reinhardtii genome had 8% and 15% AS for all and multi-exon genes respectively. The number of genes decreased precipitously as the number of AS per gene (NAG) increased in all genomes, but genomes with higher EST coverage had slower decay rate (Figure 1A). Having the highest EST coverage, A. aculeatus had the most genes with five or more NAG. However, L. bicolor with EST coverage lower than that of A. aculeatus had the largest NAG because of its higher NEG (details later). The average length of input EST affected both predicted mRNA length and fraction of AS. Short EST’s had smaller chance mapping over introns, which reduced the number of observed AS. We also observed as much as 51% of the genes having antisense, which highlighted the difficulty of gene modeling with EST.
Although fraction of AS was not affected by different sequencing technologies, we found that the relative expression levels of minor isoforms compared to major isoforms were distorted by the Solexa technology (Supplemental III; Figures S3,S4).
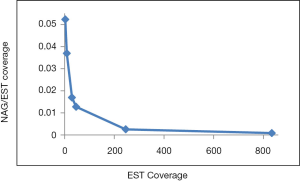
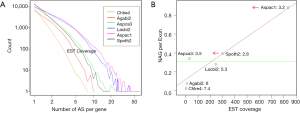
NEG, expression level, and longest intron length (MIL) determine NAG
AS is known to correlate with NEG (9). Here, we found that three independent gene features: NEG, expression level, and MIL, together, positively contributed to NAG, which can be approximated by a multivariate linear model (P values range from 0 to 6.81E-10; Table 2). Given a cellular environment, the expression level is an inherent gene feature that also depends on the means of measurement. Although the lengths of genomic and mRNA of genes also correlated with NAG when examined separately (data not shown), they were dependent on the number of exons of genes; moreover, genomic length also depended on intron length that is related to MIL. Therefore, mRNA and genomic lengths were not included in the linear model.
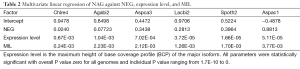
Full table
The linear coefficient for NEG (measuring increase in NAG per additional exon) correlated with the EST coverage (Figure 1B); this made intuitive sense because higher EST coverage would reveal more AS per exon. The intron-rich genomes were below the regression line; whereas, the intron-poor ones were above. The horizontal line at 0.31 (midpoint between L. bicolor and A. carbonarius) and the regression line served a divider between intron-rich and intron-poor genomes (Figure 1B). L. bicolor (57 nt average EST length) and S. thermophile (37 nt average EST length) had very similar EST coverage, but the shorter input EST length of S. thermophile made the EST coverage less effective (the data point should be further above the regression line). The effective EST coverage was also lower at very high EST coverage because of the saturation effect (Figure S2); therefore, A. aculeatus should have been further above the regression line. The results here suggested that intron-rich genomes suppressed AS.
The coefficient for expression level measures the change of NAG per expression level. This parameter was mainly dependent on sequencing platform; coefficients derived from non-Solexa platform were about 10 fold higher: C. reinhardtii (6.65E-4), A. bisporus (1.04E-03) and A. carbonarius (7.02E-04) compared to those derived Solexa platform (1.66E-05 to 5.11E-05). Solexa technology under represent minor isoforms (Supplemental III; Figure S3), and it may distort relative express of different genes.
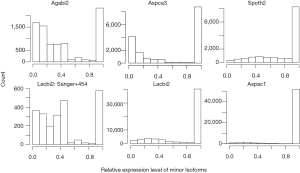
The coefficients for MIL, measuring the change of NAG per nucleotide from the longest intron of each gene, were similar in different fungal genomes and ranged from 1.26E-03 for L. bicolor to 3.77E-03 for A. aculeatus. This coefficient is about 10 fold smaller for C. reinhardtii owing to its much longer average intron length (about 5× longer) compared to fungal genomes. This means that long introns in genomes with average short introns are more predictive of AS.
The relationship between NAG and the three independent variables was more complicated than a simple linear relationship (Supplemental IV; Figures S5-S10). AS suppression in intron-rich genes within the same genome was evident by the much higher slope for NAG vs. NEG at the lower NEG compare to higher NEG. On the contrary, NAG showed a pure linear relationship with log-transformed expression levels. There were also very few data points for any extremely large predictor values (smaller sample size) making their influence less important to the overall statistics.
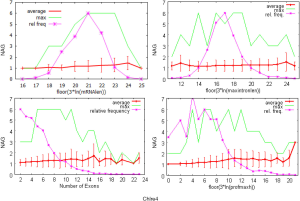
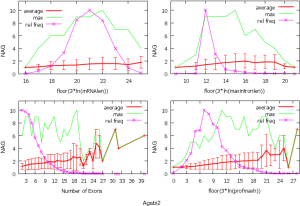
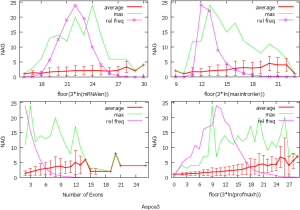
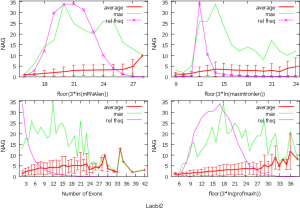
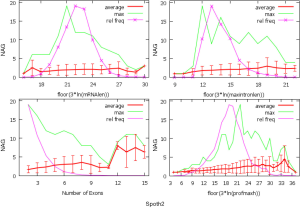
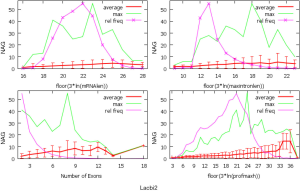
Alternatively spliced genes are more ancient
Irimia et al. (9) have shown that ancient genes, based on KOG, are more likely to have AS. Here, we further probed this subject with a different definition for ancientness: existence of orthologs in three domains of life (Archaea, Bacteria, and Eukaryotes; see methods for detail). The AS proteins compared to non-AS proteins from all five genomes had statistically significant higher proportion of hits to all three domains of life (Figure 2). In addition, we manually examined 100 genes from A. carbonarius with the most number of AS, and their proteins were enriched in ancient proteins (data not shown). The top 10 were: glyceraldehyde-3-phosphate dehydrogenase, NAD-dependent malate dehydrogenase, zinc knuckle domain protein, 60S ribosomal protein L13, L30, and L35, extracellular alpha-amylase, conserved hypothetical protein, uncharacterized peptide, and nucleosome-binding protein (Nhp6a).
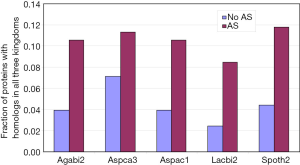
AS is conserved between fungal genomes
The ancient nature of AS implies its conservation. The pattern of AS is not conserved between human and mouse or rat (23) but is conserved between human and chimpanzee in majority of genes (24). Similarly, 92% of CEs are conserved among three Caenorhabditis species (25). As the first step towards understanding the conservation of AS, we surveyed the distribution of AS types and found sparse CE among fungal genomes: 1.1-2.5% (2.1-6.7% if including variant of CE) of AS; whereas, C. reinhardtii has 8.2% CE (12% if including variants of CE). Majority of fungal and green algal AS was RI (50-78%), followed by AA 11-23% and AD 6-15% (Supplemental V; Figure S11). AS conservation was measured by comparing expected and observed fractions of orthologous gene pairs in terms of AS and NoAS: NoAS/NoAS, NoAS/AS, AS/NoAS, and AS/AS (Figure 3A). There was a consistent over representation of observed AS/AS pairs compared to expectation with P values <2.2E-16 for all pairs of fungal genomes; this over representation was the smallest for A. bisporus/L. bicolor (0.16) and largest for A. aculeatus/L. bicolor 0.43. The smaller fractions of AS/AS pairs involving A. bisporus were due to its lower EST coverage that under estimated true AS. The four representative pairs of genomes (total 10 combination out of 5 genomes) in Figure 3 spanned different evolutionary distances: the A. carbonarius/A. aculeatus pair belonged to the same genus, the two pairs S. thermophile/A. aculeatus and A. bisporus/L. bicolor were from Ascomycota and Basidiomycota respectively (at phylum level), and the A. aculeatus/L. bicolor pair were from different phyla. Inter phyla AS conservation was not less than that at genus level.
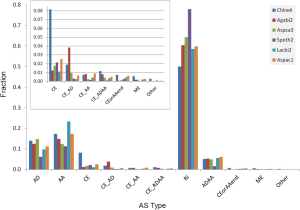
Detailed analysis of conservation of AS profile is a very labor-intensive endeavor (26), so we used an exon-alignment algorithm (unpublished from orpara.com; see Methods for detail) to classify the conservation of exon structure between a pairs of orthologous genes into five categories: perfect, near perfect, indel, partial, and none. Owing to the lack of conservation of UTR exons between orthologous gene pairs in fungi (data not shown), we used only coding exons in our analysis. The fraction of gene pairs with any structural conservation (combination of perfect, near perfect, indel, and partial) is shown in Figure 3B. The AS/AS gene pairs showed the highest fraction of exon structure conservation compared with AS/NOAS, NOAS/AS, or NOAS/NOAS. The detailed break-down for exon structure conservation from the comparison of all possible pairs of gene models from an orthologous gene pair shifted from more perfect to more partial conservation as the evolutionary distance between genomes increased (Figure 3C). The two Aspergillus species had the most perfect (48%) and near-perfect (26%) exon structure conservation. Above genus level, however, exon alignments were dominated by partial exon alignments. The exon alignment in the AS/AS category from Basidiomycota genomes A. bisporus/L. bicolor showed more perfect (19%) and near-perfect alignments (18%) than those (7% and 10%) from Ascomycota genomes S. thermophile/A. carbonarius. This is consistent with more intron loss in Ascomycota (27). The exon alignment between phyla represented by A. aculeatus/L. bicolor contained very little perfect (2%) and near-perfect (5%) type, and was dominated by partial alignments (54%) in the AS/AS category. The level of conservation above genus and at phylum levels (counting perfect alignments) was comparable to the number of conserved CE [390] in closely related species of Caenorhabditis (9).
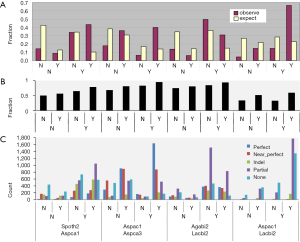
Coding region, stop codon, and RI type dictate intron 3n length bias
The splicing machinery is error prone and relies on nonsense-mediated mRNA decay (NMD) to eliminate aberrant splicing products (20,28). NMD should be essential for fungal genomes with high fractions of RI (Table 1 and Figure S4) that in turn had the potential to generate harmful truncations or insertions in proteins. One strategy for the genome to mitigate the deleterious effects of unintended RI is to avoid 3n intron length or to evolve stop codons within 3n introns. This will trigger NMD in the event of unintended RI when such introns are not located near the 3’ end of the mRNA. To evaluate how 3n and stop codon play a role in evolution, we classified introns into major RI (retained in the major isoform), minor RI (retained in a minor isoform), and NRI (retention not detected). The minor RI introns were noticeably shorter than both NRI and major RI introns (Supplemental VI; Table S1).
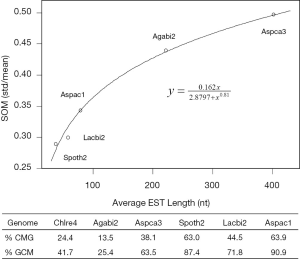
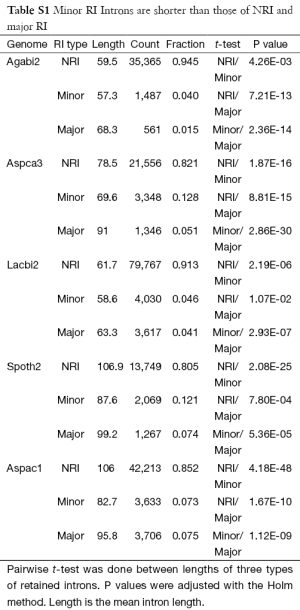
Full table
If the intron length were randomly distributed, then the 3n, 3n+1, and 3n+2 lengths should be uniformly distributed. Two genomes, A. aculeatus and C. reinhardtii had 1/3 uniform distribution, whereas the other four genomes avoided 3n introns (Figure 4A). In the direction of increasing EST coverage, the P values for chi-square test against uniform distribution were 0.84, 5.2E-09, 3.3E-06, 2.6E-05, 0.0017, and 0.33, respectively, for C. reinhardtii, A. bisporus, A. carbonarius, L. bicolor, S. thermophile, and A. aculeatus. The difference of 3n avoidance among fungal genomes could be explained by the segregation of 3n, 3n+1, and 3n+2 introns among genes or isoforms of different expression levels. At lower EST coverage, introns were enriched in moderate to highly expressed genes or isoforms that avoided 3n length. At higher EST coverage, introns from ever decreasing levels of expression will accumulate, thus leading to uniform distribution of 3n, 3n+1, and 3n+2 intron length. This view was supported by more detailed analysis of intron 3n avoidance of highly expressed major isoforms to all introns (Supplemental VII; Figures S12,S13). The lack of whole genome level 3n avoidance for C. reinhardtii is due to its five times longer average intron length when compared to introns of fungal genomes.
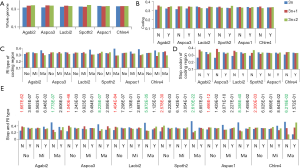
Because RI of 3n introns has potential impact on protein structure, we limit our focus on coding regions. Introns from coding regions either maintained (in lower EST coverage genomes A. bisporus and A. carbonarius) or enhanced (in higher EST coverage genomes L. bicolor and S. thermophile) the 3n deficit pattern (Figure 4B). For A. aculeatus (the highest EST coverage) and C. reinhardtii (long introns), no 3n avoidance was seen in the coding introns. Noticeably, coding introns were shorter than non-coding introns (Supplemental VIII). Within coding regions, NRI and major RI showed opposite 3n bias (Figure 4C). NRI type displayed significant 3n avoidance for all but A. aculeatus (small 3n bias with P value 0.13) and C. reinhardtii (no 3n bias, P value 0.79). Whereas the major RI from coding regions showed enrichment of 3n length (Figure 4C); however, the P values of Chi-square test against 1/3 from major RI were insignificant for A. bisporus (0.076; favor 3n preference but not overwhelming), A. carbonarius (0.36), C. reinhardtii (0.72; due to smaller counts). Within coding regions, introns with stop codons showed no 3n deficit in all genomes, whereas stopless introns avoided 3n more markedly except for A. aculeatus (Figure 4D).
Despite the weaker trend of stop codon and RI type when considered separately, we found consistent 3n bias across all genomes when combining these two factors (Figure 4E; P values from Chi-square test for C. reinhardtii were the least significant due to the least major RI counts 47; but multinomial test of 14:9:4 on 1/3 gave probability of 1.88E-03). Stopless NRI introns avoided 3n; in contrast, stopless major RI introns preferred 3n. Stopless minor RI introns from A. aculeatus resembled major RI with 3n preference (P value 9.1E-10), but they resembled NRI in A. carbonarius with avoidance of 3n length (P value 0.0099). All other genomes had little bias. The opposite 3n bias in stopless minor RI introns in two genomes from the same genus paralleled the distorted minor isoform distribution by the Solexa EST technology and EST coverage. The fraction of stopless introns increased from NRI, minor RI, to major RI with small variation between genomes. The percentages of major RI with respect to all introns in coding regions were 0.02%, 1.1%, 4.2%, 3.2%, 6.8%, and 7.0% for C. reinhardtii, A. bisporus, A. carbonarius, L. bicolor, S. thermophile, and A. aculeatus respectively; this paralleled EST coverage. Moreover, the suppression of AS in intron-rich genomes could be seen here; L. bicolor being intron-rich and having higher EST coverage had lower fraction of both minor and major RI compared to A. carbonarius. The numbers of major RI introns that were 3n and stopless (candidates of RI with gained coding function) were 47 (0.06%), 116 (0.33%), 204 (0.92%), 702 (0.97%), 278 (1.87%) and 690 (2.04%), respectively, for C. reinhardtii, A. bisporus, A. carbonarius, L. bicolor, S. thermophile, and A. aculeatus (Numbers in parentheses are percent with respect to introns in the coding region). Within major RI, the percentages of stopless 3n introns were less variable between genomes: 29.8%, 30.9%, 22.0%, 30.7%, 27.3%, and 29.2% for the six genomes respectively. RI 3n introns without stop codons could introduce structural variations in the interior of the protein by inserting short peptides.
The divergent 3n tendency for three RI types of stopless introns from fungi could be graphically demonstrated in the peak length region (Figure 5). Furthermore, stopless major RI introns were significantly longer than both stopless NRI and minor RI introns.
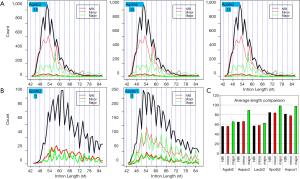
Intron phase bias and implication for protein structure evolution
The well-known phase 0 intron preference (29-31) was obvious in all genomes used in this study (P value from Chi-square test against uniform distribution ranges from 0 to 1.0E-49) (Figure 6A). Interestingly, phase 0 and 1 (to a lesser extent) introns were strongly favored in stopless introns (28-38% of coding introns) as opposed to stop-containing introns that showed little phase 0 preference (Figure 6B). Major RI introns from all genomes (C. reinhardtii multinomial exact test against stopless population had probability 0.0218) and minor RI from four genomes showed avoidance of phase 0. Major RI from Basidiomycota genomes preferred phase 1, and major RI from Ascomycota genomes favored phase 2 in the background of stopless introns (Figure 6C). Minor RI from A. bisporus, S. thermophile, A. aculeatus, and C. reinhardtii also preferred phase 1 against the background of stopless introns (Figure 6C). Of the stopless introns, 3n intron favored phase 1 and avoided phase 0; this pattern was weak for A. aculeatus and tiny for C. reinhardtii (probabilities of multinomial test against stopless population were 1.84E-04 and 7.64E-04 respectively) (Figure 6D).
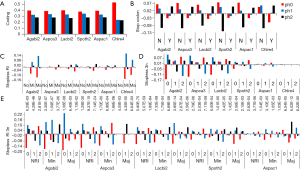
Next, we examined the combined effect of 3n length and RI types on phase distribution and found that the two Basidiomycota genomes L. bicolor and A. bisporus had similar patterns: 3n intron in all RI categories favored phase 1 and avoided phase 0 compared to the stopless intron population (very low P values; Figure 6E). The three Ascomycota genomes had similar patterns of favoring phase 1 and avoiding phase 0 in NRI and minor RI 3n introns. C. reinhardtii showed phase 0 avoidance and phase 1 and 2 preference in minor RI introns only; unfortunately, the counts were too low to be statistically significant (data not shown). Phase 2 preference was observed for 3n+2 minor RI of A. bisporus, 3n+1 major RI of A. carbonarius, 3n+2 minor RI of L. bicolor, 3n+1 major RI of S. thermophile, and 3n+1 major RI of A. aculeatus.
To gain insight into the phase bias, we listed the possible amino acids at the intron/exon junction as if stopless 3n introns were retained; none 3n introns would cause frame-shift in the 3’ intron bounds as illustrated for 3n+1 and 3n+2 introns (Figure 7). The almost invariant GT.AG (96.7-99.6% in this study; Supplemental VII) and other conserved nucleotides in fungi (excluding S. cerevisiae) (32) were shown in Figure 7. The consensus of fungi has an extra G in the 5’-end of exon and T before the 3’-conserved YAG compared to that of a broad spectrum of eukaryotes (33-35). We tabulated both the observed and expected (based on observed base frequency) fractions of stop codon-free (stopless) splicing boundaries involving consensus bases (Figure 7) based on fungal genomes used in this study. There were tree codons in the 5’ bounds for all phases. For the 3’ bounds, phase 0 and 2 had two codons, and phase 1 had three codons. The observed fractions of stopless 5’-intron bounds were 0.96, 0.91, and 0.54 for phase 0, 1, and 2 respectively; those for the 3’-bounds (assuming 3n introns) were 0.61, 0.95, and 0.98 respectively. In 3n introns, the probability of stopless translation for both intron bounds combined was the product of the two probabilities for 5’ and 3’ bounds; they were 0.58, 0.86, and 0.53 for phase 0, 1, and 2 respectively. There was a consistent, albeit small, underrepresentation (binomial test were all significant) of stop codons at the intron bounds for all phases when compared to calculations based on observed base frequencies at the intron bounds (3 nt from exon, and 9 nt from intron). The coding potential at intron bounds was greatly reduced by the consensus constraint on the bases because of the significantly lower stopless fraction when compared to random codons of uniform base frequency (0.9981 or 0.9985 for 5 or 6 codons). Because the combination of intron 3n length and intron phase determined the frame of translation at the 3’ intron bound, the fraction of stopless codons at the 5’ intron bound is the dominant factor for controlling the coding potential for different intron phases; the stopless fraction at the 5’ intron bound coincided with intron phase bias: phase 0 > phase 1 > phase 2. This tempted us to conclude that the phase bias is due to the coding potential at the 5’ intron bound as exemplified by the dramatic avoidance of phase 2 introns in stopless introns (Figure 5B). This can be understood as the 5’-located consensus stop codon in phase 2 blocks the coding potential in the event of either RI or AD (the variant extending into the intron). Historically, phase 0 might be favored during the ancient intron birth process (29,36,37).
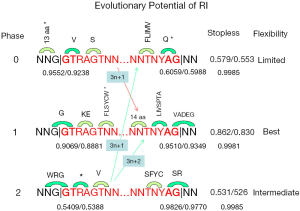
The preference of phase 1 and to a lesser extent phase 2 over phase 0 in stopless 3n introns when compared to the stopless intron population that was already phase 0 dominated (Figure 6D) could be explained by the most favorable amino acid sequences in the intron bounds of phase 1 for accommodating peptide insertion in the event of RI, by virtue of the most flexible glycine residue in its 5’ and 3’ bounds. Phase 2 had glycine only in the 5’ intron bound, whereas phase 0 had none. In phase 0, the 5’ splice site was dominated by valine (hydrophobic and bulky) and serine (hydrophilic and flexible), and the 3’ splice site permitted hydrophobic and bulky residues followed by glutamine. In phase 1, the 5’ splice site allowed glycine followed by charged residues lysine or glutamic acid; on the 3’ splice site, seven possible amino acids (L, I, V, S, P, T, and A) were followed by five possible amino acids V, A, D, E, and G (except for valine, these were flexible and hydrophilic or charged). The greater choice of amino acids on both ends of phase 1 (higher entropy) meant higher evolutionary potential. Better yet, codon preference/avoidance (38) also favored phase 1. The 5’ splice site of phase 2 started with W, R, or G followed by a consensus stop codon and valine; most of them were bulky and hydrophobic. The 3’ splice site of phase 2 had S, F, Y, or C followed serine and arginine that was disfavored in proteins (39).
The preference of phase 2 by none 3n major or minor RI stopless introns (Figure 6E) could be explained by favorable glycine in the 5’ bound of this phase and the combination of 3’ bound of phase 0 (3n+1) and phase 1 (3n+2). The unfavorable 3’ bound of phase 2 (especially the unfavorable arginine) could be avoided in such arrangements. This class of AS would create alternative C-terminal ends as appose to inserting peptides for stopless 3n introns. For the major RI stopless introns, the intron-rich Basidiomycota genomes were dominated by phase 0 deficiency and phase 1 excess in stopless 3n introns; whereas, the intron-poor Ascomycota genomes were dominated by 3n+1 introns with phase 0 (3n+1 introns combine 5’ phase 0 to 3’ phase 1) deficiency and phase 2 (3n+1 introns combine 5’ phase 2 to 3’ phase 0) excess. This demonstrated that Ascomycota genomes were dominated by C-terminal variation through RI, in contrast to peptide insertion in the middle of protein in Basidiomycota genomes.
Discussion
We analyzed AS of one green algal and five fungal genomes with varying degree of EST coverage using a novel EST-based gene modeling algorithm (COMBEST) that also yields relative expression levels of alternatively spliced isoforms for each gene. Partial EST models and ab initio gene predictions are excluded in our analysis. Solexa technology tends to diminish the proportion of minor isoforms with relatively low expression levels compared to Sanger and 454 without affecting intergenic expression variations (Supplemental III). Nonetheless, aided by the COMBEST algorithm, we revealed abundant AS in fungal genomes, the stochastic nature of AS, and its role in evolution. The ancientness of AS is also reveled in this study. Fungal genes with AS, compared to those without AS, have significantly higher fractions of proteins with homologs in all three domains of life; this is consistent with the ancient nature of AS discovered independently from three animal and one plant genomes (9). The ancientness of AS has also been demonstrated by the conservation of CE of four genes from diverse eukaryotes (40). In this study, pure CE ranges from 1.1% to 2.5% in fungal genome and 8.2% in the green algae genome. The combined CE and its variant can reach 6.7% in fungal genomes and 12% in the green algal genome. It would be interesting to see how many of these are conserved in animal and plant genomes. As expected, AS is conserved between fungal genomes from different phyla; furthermore, the highest exon structure conservation in AS/AS orthologous pairs indicates that AS profile were also conserved (Figure 3).
The high fraction of AS is an indication of stochastic nature of splicing
The stochastic nature of splicing (41) implies that a given AS isoform (regardless of cellular function) will be observed once sufficient number of mRNA is sampled (42,43). The positive correlation between NAG and expression level (Table 2) illustrates this point. Manual examination of BCP of alternatively spliced forms from A. carbonarius, and the distribution of relative expression of minor isoforms from Sanger and 454 technologies (less distorted minor isoforms; Figure S3) reveal that many minor isoforms are expressed at very low levels compared to the major isoforms. The marginally expressed isoforms are more likely to be a consequence of splicing error than an intended biological function. The suppression of AS by both intron-rich genomes and intron-rich genes within the same genome is consistent with the low error rates in intron-rich or highly expressed genes (42).
Because different eukaryotic genomes, including different fungal genomes, differ in the fractions of multiexon genes, the fraction of AS in multiexon genes is a more meaningful measure of the extent of AS. Owing to very limited EST coverage, we only see 15% of AS in the multiexon genes from C. reinhardtii. Not surprisingly, up to 60% of plant intron-containing genes have AS (44). In fungal genomes, the actual AS in multiexon genes should be higher than 73%, the highest we see in this study, owing to the positive relationship between observable AS and EST coverage even though rewards for additional EST coverage diminishes. Accordingly, 76% multiexon genes from Trichoderma longibrachiatum, an Ascomycota genome, have AS when interrogated with high EST coverage and different algorithms (8). At high EST coverage, over 90% of genes have overlapping transcription (Figure S1) making EST-based gene-modeling extremely challenging; the COMBEST algorithm overcomes this difficulty. Additionally, over 50% of the genes have antisense transcription given enough EST coverage (Table 1). Higher EST coverage reveals more AS, overlapping transcription, and antisense transcription. This paints a picture of rampant transcription and error-prone splicing.
It is not surprising that previous reported AS is much lower because of lower EST coverage (6,7,45). Mekouar et al. reported 9.2% AS, also dominated by RI, in multiexon genes from Yarrowia lipolytica genome based on 28,434 EST sequences (45). The length of consensus splice site from Y. lipolytica is in between those of intron-rich genomes and intron-poor ones exemplified by Sacchromyces cerevisiae that has only 250 introns. Moreover, 3n stopless introns are strongly suppressed, and majority of AS are non-functional in the Y. lipolytica genome (45). Intensive investigations of the S. cerevisiae genome have revealed several functional AS: thirteen cases of transcript abundance regulation of meiosis-specific genes (46-48), auto-regulation of splicing factors RPL30 (49) and YRA1Jeny (50-52), and two cases of protein diversity, SRC1 (53) and PTC7 (54). PTC7 achieves protein diversity through RI of a stopless 3n intron. It seems that this intron-poor genome still has at least 7% AS of multiexon genes and most of them are functional.
The above seems to conform to the trend toward lengthening of consensus sequences for splicing and more precise splicing when genomes become intron-poor (33,34,55); meanwhile, AS gets less frequent with a concomitant increate of the fraction of functional AS. In another word, splicing is stochastic for intron-rich genomes and deterministic for intron-poor genomes. Intron-rich genomes rely on NMD for eliminating incorrectly spliced transcripts. Therefore, only a small fraction of AS should be functional in intron-rich genomes. There is no simple numeric measure for functional AS, but fraction of major RI (7%) may serve as a surrogate because RI is the dominant form of AS in fungi. Detecting peptides that spans AS boundaries is probably the most conservative estimate of functional AS that is sizeable in fungal genomes (56). Regardless, fraction of 3n and stopless introns involved in major or minor RI are prime candidates for RI-mediated protein structure diversity. The lower limit is major RI only (0.062-2.0%), and the upper limit is minor plus major RI (0.128-3.4%). Therefore, up to 2-3% of the introns may have functional RI. The percentage of stopless 3n introns within each RI category is significantly larger: minor RI 6.2-16.3% and major RI 22-31%. The NRI introns range from 92% to 96% for Basidiomycota and 80% to 86% for Ascomycota genomes; this is also consistent with suppression of, potentially erroneous, AS in intron-rich genomes.
Extent of AS and its suppression
The extent of observable AS depends on both the depth of EST coverage and the innate property of genomes. Clearly NEG, expression levels, and MIL together determine NAG, and not the other way around. Previous studies have noticed the connection between AS and intron number (9) or intron length (57) individually. For a fixed probability of AS per intron, genes with more introns will have more AS. Higher expression of a gene gives more opportunity to sample AS through EST. The major RI introns (proxy for functional RI) are longer (Figure 5). Alternatively spliced introns tend to contain regulatory elements and thus longer. Moreover, CE by definition requires long introns to contain alternative exons.
Although NAG positively correlates with NEG, intron-rich genomes and intron-genes from the same genome tune down AS. The suppression of AS is desirable because majority AS are non-functional. The most likely outcome of AS for short introns is non-functional RI; fortunately, short introns prefer none 3n length, which triggers NMD when retained. This will result in less AS for short introns. Long introns are more likely to be involved in functional AS; the predominantly short introns in fungal genomes might be linked to AS suppression by means of more efficient splicing through the intron definition pathway of splicing (58,59).
Intron phase enigma and bridging function of AS in evolution
The fact that genome-wide 3n avoidance only appears in genomes with low-EST coverage indicates that 3n introns are not evolutionarily selected against, but there is a mechanism (either by evolutionary selection or by regulation) other than NMD that reduces the abundance of transcripts containing 3n stopless introns. In A. aculeatus genome, 3n intron bias was only observed in two of the six partitions (three RI types x stop/stopless; Figure 4E). This suggests that stopless 3n introns evolve into major RI through an intermediate state of minor RI (Figure 8). The prominent 3n deficiency in stopless NRI introns of genomes with lower EST coverage is presumably due to low abundance of transcripts containing 3n stopless introns. In contract, the fraction of 3n length in stopless major RI introns are more or less the same, around 50% (compare to 33% uniform distribution), in different genomes. Abundant transcripts containing NRI stopless 3n introns must efficiently splice out these introns, which may rely on the concerted evolution of the spliceosome. The reason for lack of selection to eliminate 3n stopless introns might be that such introns are raw materials for evolving new coding functions and thus are beneficial.
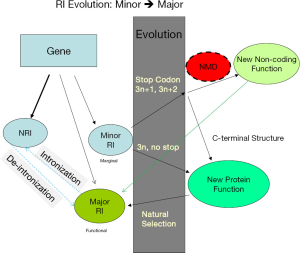
The phase 0 preference is universal in eukaryotes (29), in addition both introns and AS are ancient (9,40,55,60). Therefore, phase bias is likely present in the ancestor of eukaryotes. Stopless as opposed to stop-containing introns have drastically more phase 0 preference (Figure 6A,B); this indicates a coding function for introns. The phase bias enigma has never been satisfactorily solved (61,62), and we propose its possible link to AS and the fraction of stopless 5’ intron bound from three different phases: 0>1>2 (Figure 7). This is based on the constrained coding capacity (63,64) of intron bounds when involved in RI and AD (extending into introns) and the bridging function of introns in evolution. The tolerance of protein structure toward insertion/deletion of structural elements (65) through flexible and hydrophilic links can partially explain the phase bias observed when RI is involved. Phase 0 intron tends to be associated with helix and coil, phase 1 associated with coil, and phase 2 is evenly distributed among helix, coil and sheets (66). Insertion/deletion of structural elements through RI, AD, and AA would be more favorable in phase 1. The 3’ bound is less important in phase discrimination because of its less sequence constraint compared to that of the 5’, and its reading frame is determined by the 3n length of the stopless intron; however, when stopless 3n AA is involved, analogous to RI of stopless 3n intron, phase 1 is preferred (67). Historically, ancient gene birth through exon-shuffling favors phase 0 (29,68-70). Both ancient introns and exons, the latter about 75 nt (27) that are significantly shorter than the current average folding domain (178 aa), are much shorter than their modern counter parts. The objective at the very beginning of evolution is to construct diverse protein domains and structures, which could be facilitated by either exon-shuffling or AS (71). Phase 0 RI stopless 3n introns are bordered by residues favoring both alpha-helix and coil, whereas phase 1 RI stopless 3n introns disfavor secondary structures. This may explain their enrichment in between domains; indeed, phase 1 appears to be prominent in forming multi-modular proteins in vertebrate evolution (72).
NMD besides being a quality control mechanism for splicing also regulates transcript abundance (73,74). RI dominates fungal and green algal AS; fortunately, most introns with potential for RI have evolved a safeguard for being targeted by NMD in the event of RI: PTC or none 3n length. Introns with such features are 90-94% for NRI introns and 83-90% for minor RI introns. The rare major stopless 3n RI introns (0.06-2%) can contribute to functional protein structural diversity through insertion of short peptides (65,75). We propose a model for how NRI, aided by a marginal (minor) RI, emerges as functional (major) RI through the evolution of novel structure and function and the reverse: intronization (Figure 8). Here major RI is slightly different from the earlier operational definition for the purpose of data analysis; the newly evolved functional RI (corresponding to major RI in data analysis) can co-exist with the ancestral isoform if both isoforms are functionally beneficial. Initially an intron has a small probability to be retained owing to the stochastic nature of splicing. By virtual of its low expression or being a target of NMD (non-3n length or containing PTC), this marginal RI will not interfere with the function of the original spliced form and could evolve through neutral drift. Over time, introns with marginal RI accumulate beneficial mutations; subsequent natural selection establishes the marginal isoforms as functional RI. However, the relative abundance of NRI and RI is determined by the optimal subcellular, spatial, and temporal requirements. The term intronization is conceived in the context of intron gain/loss (76), but it is more natural to be considered under our model: RI → (RI + NRI). However, intronization is rare owing to the greater constraint on evolving a new intron in the context of protein coding and is only detectable in the recent past (76-80). Intronization seems to be favored in UTR (81) and a subset of retrogenes where 3n stopless introns are favored (79). Furthermore, introns evolved from retrogenes are dominated by cryptic splice sites in their parent genes (79), which suggests the involvement of RI. For example, the intronization of vulcan gene involves RI (78) with the RI isoform more abundant than NRI. In this study, we could not distinguish whether an NRI/RI pair was derived from intronization or RI; however, we believe the latter to be the dominant evolutionary direction. The key idea is that AS bridges evolution of non-functional to functional coding introns as well as the reverse process of shortening a protein by converting coding sequences to introns. In support of our model, PTC7 in yeast uses RI (intron is stopless 3n, phase 1) to insert a peptide that contains a transmembrane domain for a different subcellular localization (54). There are more examples where RI contribute to alternative C-termini, and the introns either contain stop codons or are none 3n length (82-86). For instance, the Drosophila RFeSP locus evolved a new protein by natural selection through a stopless 3n+1 phase 0 RI over 250 million years (83). A novel C-terminus generated through RI of phase 2 stop codon-containing intron in an ancillary subunit of a voltage-gated sodium channel is different in density, gating and pharmacological properties (84). About 63 million years ago (MYA) inside the last intron of the ZRANB2 gene of old world monkey, a LINE element was exonized, followed by RI of the preceding phase 2 stop-containing intron (86). In the pig ANKRD1 gene, intron 6 is 3n stopless, intron 7 is none 3n, and intron 8 has an in-frame stop codon; RIs of introns 8, 7|8, and 6|8|9 respectively generate three functional variants that differ at the C-termini (82). All minor RI and NRI in the Arabidopsis Dicer-like 2 gene either are none 3n or contain stop codons (87). RI regulates mRNA abundance in mammalian genomes (88-90) whose introns are much longer. Short introns in the human genome have higher incidence of RI (91).
Our model for RI could also be applied to AD and AA where only 5’-portion and 3’-portion of the intron are involved in the alternative coding function respectively. This can be considered as RI of part of the intron. The 3n length of stopless portion of AD and AA also influences coding frame of 3’ exons in the same way as it does in RI. AD and AA are more adapted to fine-tuning of protein structure compared to RI where the whole intron is involved. The former can generate indel of a single amino acid (92) as well as indel of structural domains in genomes with long introns. The third intron in the PLP gene is 1,176 nt that is flanked by exons of 157 nt on the 5’-end and 169 nt on the 3’-end. AD of 3n stopless 105 nt (35 aa) demarks the evolution of three major branches of jawed vertebrates (93). AD of 9 bases produces two proteins of different sub-nuclear distributions (94). The phase 1 bias of AA affecting single amino acids (67) can also be explained by the avoidance of stop codon that is part of the model proposed here.
Overlapping transcription AS as a bridge for emergence of novel fusion genes
We observed significant transcript overlap between genes. Since these overlapping transcriptions happen at very low frequencies, they are not going to be a burden for the organism. But, in very rare cases, splicing of the intron between neighboring genes could produce fusion proteins (95). This process is a special variant of AS and deserves further consideration under the bridging function of AS. Genes for secondary metabolite are usually clustered in fungi (96,97) as well as in plants (98); here we will not do a full literature survey. Multi-domain proteins are common themes in fungi (99,100). It would be interesting to see whether AS plays a role in the evolution from gene cluster to multi-domain proteins.
Importance of AS in stem cells
In embryonic stem cells (ESC), reprogrammed pluripotent stem cells (PSC), or induced PSC (iPSC) AS is one of the three important regulatory mechanisms: transcript regulation, AS regulation, and miRNA (21,101-103). Stem cells need to maintain a delicate balance between self-renewal, pluripotency, differentiation, and cancer. For this transcription regulation is not sufficient, and it requires the fine-grained regulation by AS with the help of miRNA and translational control. Key regulators in stem cells are enriched in transcript factors and receptors or their ligands. AS regulation is mainly archived through CE, AD, ME, and alternative transcript start and stop are. MBNL proteins control a large program of exon inclusion/exclusion events that are differentially regulated between ESCs and other cell types (104). One of the MBNL targets Foxp1 gene is regulated through ME; in human ESC, exon 18b is included as oppose to exon 18 in differentiated cells (104,105). The master regulator of pluripotency Oct4 has 3 isoforms: Oct4A, Oct4B, and Oct4B-1 (106,107). The difference between Oct4A and Oct4B is the combination of alternative transcription start and AA of the exon 2 (108). Relative to Oct4B, Oct4B-1 is RI of intron 2 (106). However, this RI event results in truncation rather than structural modification of Oct4B. The pluripotent factor Sall4 has two isoforms A and B, with B being the AD of exon 2 (109), which shortens exon 2 (110). In mouse, TCF3 uses CE exon 5, Smarcb1 uses AD exon 2, Ctnnd1 uses alternative transcript start, and Serca2 uses alternative transcript stop inside the intron of another isoform (111). FGF4 isoform generated through exclusion of CE exon 2 is truncated and antagonizes the longer isoform in ESC self-renewal (112). Ikaros generate at least 5 isoforms through four CE exons that differ in DNA-binding specificity and subcellular location (113). The FGFR2 receptor uses two ME exons 8 (IIIb) (114) and 9 (IIIc) (115,116). Simultaneous skipping of CE exon 9 and 10 in c-MPL that are regulated by Rbm15 through transcription rate control by chromatin modification results in a protein lacking transmembrane and intra cellular domains with dominant negative effect on the full length isoform (117).
There is no known case of RI, or AA contributing protein diversity in stem cells. Whether this is due to the limitation of research methods still waits further attention. One interesting question is where the switching from predominantly RI in lower eukaryotes to CE, ME in higher eukaryotes is associated with stem cell evolution. What is the evolutionary advantage to use CE and ME over RI in stem cell? To answer these questions, our COMBEST algorithm may prove to a useful tool.
Acknowledgements
This work was started at the U.S. Department of Energy Joint Genome Institute and supported by the Office of Science of the U.S. Department of Energy under Contract No. DE-AC02-05CH11231. The work was finished with KZ’s free time after he left JGI on Dec 31, 2011 with new ideas formulated during this period. No resource from Roche Molecular Diagnostics (RMD) was used towards this work, and this work was done before KZ joined RMD. The author KZ wishes to thank Dr. Zhong Wang from JGI for his significant contribution by discussing many ideas and reviewing and criticism of this paper. We thank Sydney Brenner for his insight in the coding function of introns.
Footnote
Conflicts of Interest: The authors have no conflicts of interest to declare.
References
- Kim E, Magen A, Ast G. Different levels of alternative splicing among eukaryotes. Nucleic Acids Res 2007;35:125-31. [PubMed]
- Takeda J, Suzuki Y, Nakao M, et al. Large-scale identification and characterization of alternative splicing variants of human gene transcripts using 56,419 completely sequenced and manually annotated full-length cDNAs. Nucleic Acids Res 2006;34:3917-28. [PubMed]
- Pan Q, Shai O, Lee LJ, et al. Deep surveying of alternative splicing complexity in the human transcriptome by high-throughput sequencing. Nat Genet 2008;40:1413-5. [PubMed]
- Stolc V, Gauhar Z, Mason C, et al. A gene expression map for the euchromatic genome of Drosophila melanogaster. Science 2004;306:655-60. [PubMed]
- Campbell MA, Haas BJ, Hamilton JP, et al. Comprehensive analysis of alternative splicing in rice and comparative analyses with Arabidopsis. BMC Genomics 2006;7:327. [PubMed]
- Loftus BJ, Fung E, Roncaglia P, et al. The genome of the basidiomycetous yeast and human pathogen Cryptococcus neoformans. Science 2005;307:1321-4. [PubMed]
- Ho EC, Cahill MJ, Saville BJ. Gene discovery and transcript analyses in the corn smut pathogen Ustilago maydis: expressed sequence tag and genome sequence comparison. BMC Genomics 2007;8:334. [PubMed]
- Xie BB, Li D, Shi WL, et al. Deep RNA sequencing reveals a high frequency of alternative splicing events in the fungus Trichoderma longibrachiatum. BMC Genomics 2015;16:54. [PubMed]
- Irimia M, Rukov JL, Penny D, et al. Functional and evolutionary analysis of alternatively spliced genes is consistent with an early eukaryotic origin of alternative splicing. BMC Evol Biol 2007;7:188. [PubMed]
- Fong YW, Zhou Q. Stimulatory effect of splicing factors on transcriptional elongation. Nature. 2001;414:929-33. [PubMed]
- Masuda S, Das R, Cheng H, et al. Recruitment of the human TREX complex to mRNA during splicing. Genes Dev 2005;19:1512-7. [PubMed]
- Rigo F, Martinson HG. Functional coupling of last-intron splicing and 3'-end processing to transcription in vitro: the poly(A) signal couples to splicing before committing to cleavage. Mol Cell Biol 2008;28:849-62. [PubMed]
- Kornblihtt AR, de la Mata M, Fededa JP, et al. Multiple links between transcription and splicing. RNA 2004;10:1489-98. [PubMed]
- Furger A, O'Sullivan JM, Binnie A, et al. Promoter proximal splice sites enhance transcription. Genes Dev 2002;16:2792-9. [PubMed]
- Alexander MR, Wheatley AK, Center RJ, et al. Efficient transcription through an intron requires the binding of an Sm-type U1 snRNP with intact stem loop II to the splice donor. Nucleic Acids Res 2010;38:3041-53. [PubMed]
- Dias AP, Dufu K, Lei H, et al. A role for TREX components in the release of spliced mRNA from nuclear speckle domains. Nat Commun 2010;1:97. [PubMed]
- Kerényi Z, Mérai Z, Hiripi L, et al. Inter-kingdom conservation of mechanism of nonsense-mediated mRNA decay. EMBO J 2008;27:1585-95. [PubMed]
- Barash Y, Calarco JA, Gao W, et al. Deciphering the splicing code. Nature 2010;465:53-9. [PubMed]
- Sánchez-Sánchez F, Mittnacht S. Nonsense-mediated decay: paving the road for genome diversification. Bioessays 2008;30:926-8. [PubMed]
- Jaillon O, Bouhouche K, Gout JF, et al. Translational control of intron splicing in eukaryotes. Nature 2008;451:359-62. [PubMed]
- Chen K, Dai X, Wu J. Alternative splicing: An important mechanism in stem cell biology. World J Stem Cells 2015;7:1-10. [PubMed]
- Wu TD, Watanabe CK. GMAP: a genomic mapping and alignment program for mRNA and EST sequences. Bioinformatics 2005;21:1859-75. [PubMed]
- Modrek B, Lee CJ. Alternative splicing in the human, mouse and rat genomes is associated with an increased frequency of exon creation and/or loss. Nat Genet 2003;34:177-80. [PubMed]
- Calarco JA, Xing Y, Cáceres M, et al. Global analysis of alternative splicing differences between humans and chimpanzees. Genes Dev 2007;21:2963-75. [PubMed]
- Irimia M, Rukov JL, Penny D, et al. Widespread evolutionary conservation of alternatively spliced exons in Caenorhabditis. Mol Biol Evol 2008;25:375-82. [PubMed]
- Mudge JM, Frankish A, Fernandez-Banet J, et al. The origins, evolution, and functional potential of alternative splicing in vertebrates. Mol Biol Evol 2011;28:2949-59. [PubMed]
- Zhou K, Kuo A, Grigoriev IV. Reverse transcriptase and intron number evolution. Stem Cell Investigation 2014;1:17.
- Popp MW, Maquat LE. Organizing principles of mammalian nonsense-mediated mRNA decay. Annu Rev Genet 2013;47:139-65. [PubMed]
- Long M, Rosenberg C, Gilbert W. Intron phase correlations and the evolution of the intron/exon structure of genes. Proc Natl Acad Sci U S A 1995;92:12495-9. [PubMed]
- Smith MW. Structure of vertebrate genes: a statistical analysis implicating selection. J Mol Evol 1988;27:45-55. [PubMed]
- Fichant GA. Constraints acting on the exon positions of the splice site sequences and local amino acid composition of the protein. Hum Mol Genet 1992;1:259-67. [PubMed]
- Kupfer DM, Drabenstot SD, Buchanan KL, et al. Introns and splicing elements of five diverse fungi. Eukaryot Cell 2004;3:1088-100. [PubMed]
- Irimia M, Roy SW, Neafsey DE, et al. Complex selection on 5' splice sites in intron-rich organisms. Genome Res 2009;19:2021-7. [PubMed]
- Irimia M, Roy SW. Evolutionary convergence on highly-conserved 3' intron structures in intron-poor eukaryotes and insights into the ancestral eukaryotic genome. PLoS Genet 2008;4:e1000148. [PubMed]
- Irimia M, Penny D, Roy SW. Coevolution of genomic intron number and splice sites. Trends Genet 2007;23:321-5. [PubMed]
- Fedorov A, Roy S, Cao X, et al. Phylogenetically older introns strongly correlate with module boundaries in ancient proteins. Genome Res 2003;13:1155-7. [PubMed]
- Vibranovski MD, Sakabe NJ, de Oliveira RS, et al. Signs of ancient and modern exon-shuffling are correlated to the distribution of ancient and modern domains along proteins. J Mol Evol 2005;61:341-50. [PubMed]
- Merkl R. A survey of codon and amino acid frequency bias in microbial genomes focusing on translational efficiency. J Mol Evol 2003;57:453-66. [PubMed]
- Beals M, Gross L, Harrell S. Amino acid frequency. 1999. Available online: http://www.tiem.utk.edu/~gross/bioed/webmodules/aminoacid.htm
- Awan AR, Manfredo A, Pleiss JA. Lariat sequencing in a unicellular yeast identifies regulated alternative splicing of exons that are evolutionarily conserved with humans. Proc Natl Acad Sci U S A 2013;110:12762-7. [PubMed]
- Waks Z, Klein AM, Silver PA. Cell-to-cell variability of alternative RNA splicing. Mol Syst Biol 2011;7:506. [PubMed]
- Melamud E, Moult J. Stochastic noise in splicing machinery. Nucleic Acids Res 2009;37:4873-86. [PubMed]
- Parmley JL, Urrutia AO, Potrzebowski L, et al. Splicing and the evolution of proteins in mammals. PLoS Biol 2007;5:e14. [PubMed]
- Reddy AS, Marquez Y, Kalyna M, et al. Complexity of the alternative splicing landscape in plants. Plant Cell 2013;25:3657-83. [PubMed]
- Mekouar M, Blanc-Lenfle I, Ozanne C, et al. Detection and analysis of alternative splicing in Yarrowia lipolytica reveal structural constraints facilitating nonsense-mediated decay of intron-retaining transcripts. Genome Biol 2010;11:R65. [PubMed]
- Engebrecht JA, Voelkel-Meiman K, Roeder GS. Meiosis-specific RNA splicing in yeast. Cell 1991;66:1257-68. [PubMed]
- Nakagawa T, Ogawa H. The Saccharomyces cerevisiae MER3 gene, encoding a novel helicase-like protein, is required for crossover control in meiosis. EMBO J 1999;18:5714-23. [PubMed]
- Juneau K, Palm C, Miranda M, et al. High-density yeast-tiling array reveals previously undiscovered introns and extensive regulation of meiotic splicing. Proc Natl Acad Sci U S A 2007;104:1522-7. [PubMed]
- Vilardell J, Chartrand P, Singer RH, et al. The odyssey of a regulated transcript. RNA 2000;6:1773-80. [PubMed]
- Preker PJ, Kim KS, Guthrie C. Expression of the essential mRNA export factor Yra1p is autoregulated by a splicing-dependent mechanism. RNA 2002;8:969-80. [PubMed]
- Preker PJ, Guthrie C. Autoregulation of the mRNA export factor Yra1p requires inefficient splicing of its pre-mRNA. RNA 2006;12:994-1006. [PubMed]
- Dong S, Li C, Zenklusen D, et al. YRA1 autoregulation requires nuclear export and cytoplasmic Edc3p-mediated degradation of its pre-mRNA. Mol Cell 2007;25:559-73. [PubMed]
- Grund SE, Fischer T, Cabal GG, et al. The inner nuclear membrane protein Src1 associates with subtelomeric genes and alters their regulated gene expression. J Cell Biol 2008;182:897-910. [PubMed]
- Juneau K, Nislow C, Davis RW. Alternative splicing of PTC7 in Saccharomyces cerevisiae determines protein localization. Genetics 2009;183:185-94. [PubMed]
- Csuros M, Rogozin IB, Koonin EV. A detailed history of intron-rich eukaryotic ancestors inferred from a global survey of 100 complete genomes. PLoS Comput Biol 2011;7:e1002150. [PubMed]
- Chang KY, Georgianna DR, Heber S, et al. Detection of alternative splice variants at the proteome level in Aspergillus flavus. J Proteome Res 2010;9:1209-17. [PubMed]
- Roy M, Kim N, Xing Y, et al. The effect of intron length on exon creation ratios during the evolution of mammalian genomes. RNA 2008;14:2261-73. [PubMed]
- Will CL, Lührmann R. Spliceosome structure and function. Cold Spring Harb Perspect Biol 2011.3. [PubMed]
- Talerico M, Berget SM. Intron definition in splicing of small Drosophila introns. Mol Cell Biol 1994;14:3434-45. [PubMed]
- Roy SW, Irimia M. Splicing in the eukaryotic ancestor: form, function and dysfunction. Trends Ecol Evol 2009;24:447-55. [PubMed]
- Ruvinsky A, Eskesen ST, Eskesen FN, et al. Can codon usage bias explain intron phase distributions and exon symmetry? J Mol Evol 2005;60:99-104. [PubMed]
- Fedorov A, Suboch G, Bujakov M, et al. Analysis of nonuniformity in intron phase distribution. Nucleic Acids Res 1992;20:2553-7. [PubMed]
- Warnecke T, Parmley JL, Hurst LD. Finding exonic islands in a sea of non-coding sequence: splicing related constraints on protein composition and evolution are common in intron-rich genomes. Genome Biol 2008;9:R29. [PubMed]
- Parmley JL, Urrutia AO, Potrzebowski L, et al. Splicing and the evolution of proteins in mammals. PLoS Biol 2007;5:e14. [PubMed]
- Birzele F, Csaba G, Zimmer R. Alternative splicing and protein structure evolution. Nucleic Acids Res 2008;36:550-8. [PubMed]
- Whamond GS, Thornton JM. An analysis of intron positions in relation to nucleotides, amino acids, and protein secondary structure. J Mol Biol 2006;359:238-47. [PubMed]
- Hiller M, Huse K, Szafranski K, et al. Single-nucleotide polymorphisms in NAGNAG acceptors are highly predictive for variations of alternative splicing. Am J Hum Genet 2006;78:291-302. [PubMed]
- de Roos AD. Origins of introns based on the definition of exon modules and their conserved interfaces. Bioinformatics 2005;21:2-9. [PubMed]
- Roy SW. Recent evidence for the exon theory of genes. Genetica 2003;118:251-66. [PubMed]
- de Souza SJ, Long M, Klein RJ, et al. Toward a resolution of the introns early/late debate: only phase zero introns are correlated with the structure of ancient proteins. Proc Natl Acad Sci U S A 1998;95:5094-9. [PubMed]
- de Roos AD. Conserved intron positions in ancient protein modules. Biol Direct 2007;2:7. [PubMed]
- Liu M, Walch H, Wu S, et al. Significant expansion of exon-bordering protein domains during animal proteome evolution. Nucleic Acids Res 2005;33:95-105. [PubMed]
- Mendell JT, Sharifi NA, Meyers JL, et al. Nonsense surveillance regulates expression of diverse classes of mammalian transcripts and mutes genomic noise. Nat Genet 2004;36:1073-8. [PubMed]
- Weischenfeldt J, Waage J, Tian G, et al. Mammalian tissues defective in nonsense-mediated mRNA decay display highly aberrant splicing patterns. Genome Biol 2012;13:R35. [PubMed]
- Kim R, Guo JT. Systematic analysis of short internal indels and their impact on protein folding. BMC Struct Biol 2010;10:24. [PubMed]
- Irimia M, Rukov JL, Penny D, et al. Origin of introns by 'intronization' of exonic sequences. Trends Genet 2008;24:378-81. [PubMed]
- Zhu T, Niu DK. Mechanisms of intron loss and gain in the fission yeast Schizosaccharomyces. PLoS One 2013;8:e61683. [PubMed]
- Zhan L, Meng Q, Chen R, et al. Origin and evolution of a new retained intron on the vulcan gene in Drosophila melanogaster subgroup species. Genome 2014;57:567-72. [PubMed]
- Kang LF, Zhu ZL, Zhao Q, et al. Newly evolved introns in human retrogenes provide novel insights into their evolutionary roles. BMC Evol Biol 2012;12:128. [PubMed]
- Roy SW. Intronization, de-intronization and intron sliding are rare in Cryptococcus. BMC Evol Biol 2009;9:192. [PubMed]
- Liang X, Fomenko DE, Hua D, et al. Diversity of protein and mRNA forms of mammalian methionine sulfoxide reductase B1 due to intronization and protein processing. PLoS One 2010;5:e11497. [PubMed]
- Torrado M, Iglesias R, Nespereira B, et al. Intron retention generates ANKRD1 splice variants that are co-regulated with the main transcript in normal and failing myocardium. Gene 2009;440:28-41. [PubMed]
- Gontijo AM, Miguela V, Whiting MF, et al. Intron retention in the Drosophila melanogaster Rieske Iron Sulphur Protein gene generated a new protein. Nat Commun 2011;2:323. [PubMed]
- Bourdin CM, Moignot B, Wang L, et al. Intron retention in mRNA encoding ancillary subunit of insect voltage-gated sodium channel modulates channel expression, gating regulation and drug sensitivity. PLoS One 2013;8:e67290. [PubMed]
- Brown PJ, Kagaya R, Banham AH. Characterization of human FOXP1 isoform 2, using monoclonal antibody 4E3-G11, and intron retention as a tissue-specific mechanism generating a novel FOXP1 isoform. Histopathology 2008;52:632-7. [PubMed]
- Park SJ, Huh JW, Kim YH, et al. Intron Retention and TE Exonization Events in ZRANB2. Comp Funct Genomics 2012;2012:170208.
- He Q, Peng J, Yan F, et al. Intron retention and 3'-UTR analysis of Arabidopsis Dicer-like 2 transcripts. Mol Biol Rep 2012;39:3271-80. [PubMed]
- Yap K, Lim ZQ, Khandelia P, et al. Coordinated regulation of neuronal mRNA steady-state levels through developmentally controlled intron retention. Genes Dev 2012;26:1209-23. [PubMed]
- Wong JJ, Ritchie W, Ebner OA, et al. Orchestrated intron retention regulates normal granulocyte differentiation. Cell 2013;154:583-95. [PubMed]
- Zhang Q, Li H, Jin H, et al. The global landscape of intron retentions in lung adenocarcinoma. BMC Med Genomics 2014;7:15. [PubMed]
- Sakabe NJ, de Souza SJ. Sequence features responsible for intron retention in human. BMC Genomics 2007;8:59. [PubMed]
- Bradley RK, Merkin J, Lambert NJ, et al. Alternative splicing of RNA triplets is often regulated and accelerates proteome evolution. PLoS Biol 2012;10:e1001229. [PubMed]
- Venkatesh B, Erdmann MV, Brenner S. Molecular synapomorphies resolve evolutionary relationships of extant jawed vertebrates. Proc Natl Acad Sci U S A 2001;98:11382-7. [PubMed]
- Englert C, Vidal M, Maheswaran S, et al. Truncated WT1 mutants alter the subnuclear localization of the wild-type protein. Proc Natl Acad Sci U S A 1995;92:11960-4. [PubMed]
- Long M. A new function evolved from gene fusion. Genome Res 2000;10:1655-7. [PubMed]
- Plumridge A, Melin P, Stratford M, et al. The decarboxylation of the weak-acid preservative, sorbic acid, is encoded by linked genes in Aspergillus spp. Fungal Genet Biol 2010;47:683-92. [PubMed]
- Pel HJ, de Winde JH, Archer DB, et al. Genome sequencing and analysis of the versatile cell factory Aspergillus niger CBS 513.88. Nat Biotechnol 2007;25:221-31. [PubMed]
- Field B, Fiston-Lavier AS, Kemen A, et al. Formation of plant metabolic gene clusters within dynamic chromosomal regions. Proc Natl Acad Sci U S A 2011;108:16116-21. [PubMed]
- Hansen FT, Sørensen JL, Giese H, et al. Quick guide to polyketide synthase and nonribosomal synthetase genes in Fusarium. Int J Food Microbiol 2012;155:128-36. [PubMed]
- von Döhren H. A survey of nonribosomal peptide synthetase (NRPS) genes in Aspergillus nidulans. Fungal Genet Biol 2009;46 Suppl 1:S45-52. [PubMed]
- Kalsotra A, Cooper TA. Functional consequences of developmentally regulated alternative splicing. Nat Rev Genet 2011;12:715-29. [PubMed]
- Salomonis N, Conklin BR. Stem cell pluripotency: alternative modes of transcription regulation. Cell Cycle 2010;9:3133-4. [PubMed]
- Chepelev I, Chen X. Alternative splicing switching in stem cell lineages. Front Biol (Beijing) 2013;8:50-59. [PubMed]
- Han H, Irimia M, Ross PJ, et al. MBNL proteins repress ES-cell-specific alternative splicing and reprogramming. Nature 2013;498:241-5. [PubMed]
- Gabut M, Samavarchi-Tehrani P, Wang X, et al. An alternative splicing switch regulates embryonic stem cell pluripotency and reprogramming. Cell 2011;147:132-46. [PubMed]
- Atlasi Y, Mowla SJ, Ziaee SA, et al. OCT4 spliced variants are differentially expressed in human pluripotent and nonpluripotent cells. Stem Cells 2008;26:3068-74. [PubMed]
- Wang X, Dai J. Concise review: isoforms of OCT4 contribute to the confusing diversity in stem cell biology. Stem Cells 2010;28:885-93. [PubMed]
- Lee J, Kim HK, Rho JY, et al. The human OCT-4 isoforms differ in their ability to confer self-renewal. J Biol Chem 2006;281:33554-65. [PubMed]
- Rao S, Zhen S, Roumiantsev S, et al. Differential roles of Sall4 isoforms in embryonic stem cell pluripotency. Mol Cell Biol 2010;30:5364-80. [PubMed]
- Eildermann K, Aeckerle N, Debowski K, et al. Developmental expression of the pluripotency factor sal-like protein 4 in the monkey, human and mouse testis: restriction to premeiotic germ cells. Cells Tissues Organs 2012;196:206-20. [PubMed]
- Salomonis N, Schlieve CR, Pereira L, et al. Alternative splicing regulates mouse embryonic stem cell pluripotency and differentiation. Proc Natl Acad Sci U S A 2010;107:10514-9. [PubMed]
- Mayshar Y, Rom E, Chumakov I, et al. Fibroblast growth factor 4 and its novel splice isoform have opposing effects on the maintenance of human embryonic stem cell self-renewal. Stem Cells 2008;26:767-74. [PubMed]
- Molnár A, Georgopoulos K. The Ikaros gene encodes a family of functionally diverse zinc finger DNA-binding proteins. Mol Cell Biol 1994;14:8292-303. [PubMed]
- Guasti L, Candy Sze WC, McKay T, et al. FGF signalling through Fgfr2 isoform IIIb regulates adrenal cortex development. Mol Cell Endocrinol 2013;371:182-8. [PubMed]
- Ornitz DM, Xu J, Colvin JS, et al. Receptor specificity of the fibroblast growth factor family. J Biol Chem 1996;271:15292-7. [PubMed]
- Zhang X, Ibrahimi OA, Olsen SK, et al. Receptor specificity of the fibroblast growth factor family. The complete mammalian FGF family. J Biol Chem 2006;281:15694-700. [PubMed]
- Xiao N, Laha S, Das SP, et al. Ott1 (Rbm15) regulates thrombopoietin response in hematopoietic stem cells through alternative splicing of c-Mpl. Blood 2015;125:941-8. [PubMed]
Cite this article as: Zhou K, Salamov A, Kuo A, Aerts AL, Kong X, Grigoriev IV. Alternative splicing acting as a bridge in evolution. Stem Cell Investig 2015;2:19.